Hello, I’m Grant Glass, a unique blend of data scientist and literature enthusiast with a Ph.D. in English and Comparative Literature. My research bridges the gap between the technical and the literary worlds, allowing me to leverage machine learning and large language models to evaluate technical documentation in a technology company.
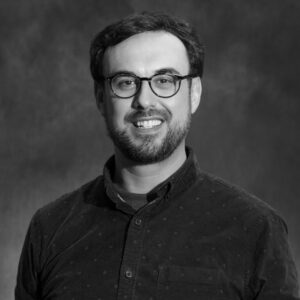
With a deep-rooted passion for both data science and literature, I’ve dedicated my career to exploring the intersection of these fields. My work involves using advanced machine learning techniques to analyze and interpret complex technical documents, enhancing their clarity and utility.
Academic Pursuits
My academic research focuses on tracing the movements of classic literary texts across time. By employing natural language processing and machine learning methodologies, I uncover patterns and insights that shed new light on historical and cultural trends in literature.
Professional Experience
In my professional role, I:
- Lead Projects: I spearhead initiatives that combine machine learning with technical writing, aiming to streamline and improve documentation processes. This involves identifying key areas where AI can enhance clarity and efficiency, and overseeing the implementation of these technologies.
- Develop Innovative Tools: I design and build tools that revolutionize document evaluation, making it easier to assess and improve technical documentation. These tools utilize machine learning algorithms to provide in-depth analysis and feedback, ensuring high standards are met consistently.
- Collaborate Across Teams: I work closely with diverse teams, including engineers, writers, and data analysts, to foster a collaborative environment. This cross-functional teamwork enhances data-driven decision-making, ensuring that projects benefit from a wide range of expertise and perspectives.
Publications & Contributions
I have published numerous papers and articles that highlight my interdisciplinary approach. Some of my notable works include:
- Fidelity, Remix, and the Adaptive Potential: Leveraging Ai and Ml Techniques In Literary Adaptation Theory. 2024. https://doi.org/10.17615/pkpv-p313
- Sussman, C., Landels, T., Bradley, I., Desir, K., Glass, G., Lewis-Meeks, A., & Harwell, J. (2023). “Died a small boy”: Re-Centering the Human in Geospatial Data from the Middle Passage. Archipelagos, 7.
- Glass, Grant. “Disruptive Reading: Resistance to Digitalization in Laurence Stern’s Tristram Shandy and Jonathan Safran Foer’s Tree of Codes.”University of California at Berkeley Comparative Literature Undergraduate Journal.
For a full list of my publications, please visit my CV page.
Personal Interests
Beyond my professional life, I enjoy:
- Reading: Delving into both classic and contemporary literature.
- Writing: Crafting essays and articles that reflect my diverse interests.
- Coding: Exploring new possibilities with computation.
Get in Touch
I’m always eager to connect with fellow enthusiasts and professionals. Feel free to reach out to me at grantglass@post.harvard.edu.